We conducted an evaluation of several models across three distinct categories that may handle multimodal long contexts. All models used greedy decoding to generate answers, with a designated generation length between 1 and 512. All evaluations were performed in a zero-shot setting. When the input length exceeds the maximum context length of the model, we keep the instruction, and truncate the interleaved image-text question from left so as to keep the question of a sample, as instruction and question are critical information and the importance of the last image is higher in many tasks. Metrics for each dataset were consistent with the original work for tasks built on previous datasets.
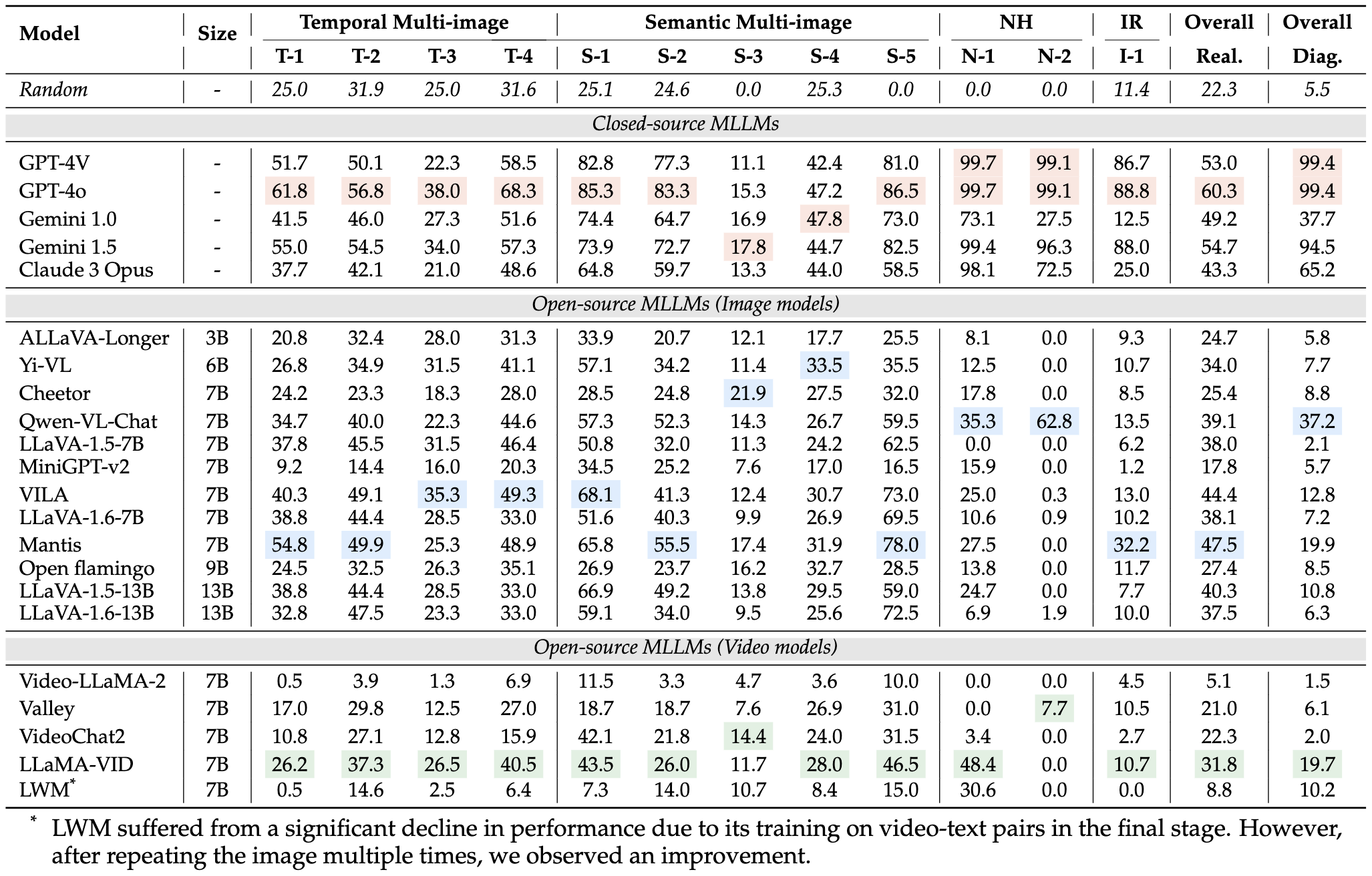
Experiment Result on MileBench. T-1 refers to the task number. NH and IR refers to Needle in a Haystack and Image Retrieval. The highest scores for closed-source models, open-source image models, and open-source video models are marked in red, blue, and green respectively.
Takeaways:
(1) Closed-source MLLMs outperform open-source MLLMs in multimodal long-context tasks.
(2) Open-source image models generally perform better than video models.
(3) The ability to adapt to long-context and perform long-context tasks are not necessarily linked.
(4) Interestingly, the majority of open-source models failed to score in the Image Needle in a Haystack task.